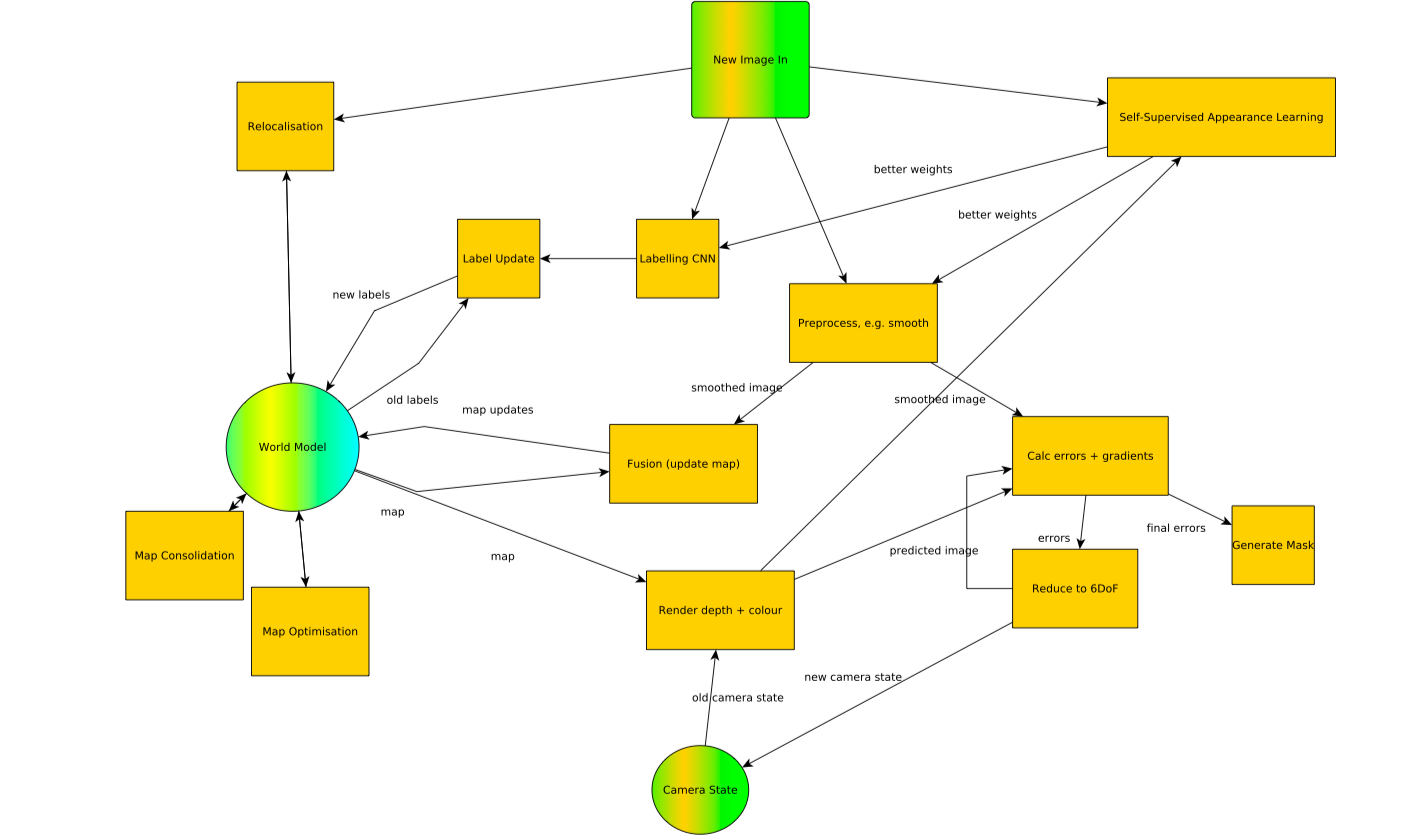
Some elements of the Spatial AI real-time computation graph. Click image to enlarge. Credit: SLAMcore
Simultaneous localization and mapping (SLAM) is the computational problem of constructing or updating a map of an unknown environment while simultaneously keeping track of a robot’s location within it. SLAM is being gradually developed towards Spatial AI, the common sense spatial reasoning that will enable robots and other artificial devices to operate in general ways in their environments.
This will enable robots to not just localize and build geometric maps, but actually interact intelligently with scenes and objects.
Enabling semantic meaning
A key technology that is helping this progress is deep learning, which has enabled many recent breakthroughs in computer vision and other areas of AI. In the context of Spatial AI, deep learning has most obviously had a big impact on bringing semantic meaning to geometric maps of the world.
Convolutional neural networks (CNNs) trained to semantically segment images or volumes have been used in research systems to label geometric reconstructions in a dense, element-by-element manner. Networks like Mask-RCNN, which detect precise object instances in images, have been demonstrated in systems that reconstruct explicit maps of static or moving 3D objects.
Deep learning vs. estimation
In these approaches, the divide between deep learning methods for semantics and hand-designed estimation methods for geometrical estimation is clear. More remarkable, at least to those of us from an estimation background, has been the emergence of learning techniques that now offer promising solutions to geometrical estimation problems. Networks can be trained to predict robust frame-to-frame visual odometry; dense optical flow prediction; or depth prediction from a single image.
When compared to hand-designed methods for the same tasks, these methods are strong on robustness, since they will always make predictions that are similar to real scenarios present in their training data. But designed methods still often have advantages in flexibility in a range of unforeseen scenarios, and in final accuracy due to the use of precise iterative optimization.
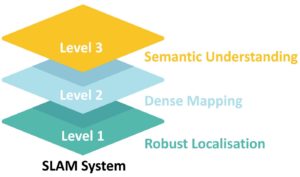
The three levels of SLAM, according to SLAMcore. Credit: SLAMcore”
The role of modular design
It is clear that Spatial AI will make increasingly strong use of deep learning methods, but an excellent question is whether we will eventually deploy systems where a single deep network trained end to end implements the whole of Spatial AI. While this is possible in principle, we believe that this is a very long-term path and that there is much more potential in the coming years to consider systems with modular combinations of designed and learned techniques.
There is an almost continuous sliding scale of possible ways to formulate such modular systems. The end-to-end learning approach is ‘pure’ in the sense that it makes minimum assumptions about the representation and computation that the system needs to complete its tasks. Deep learning is free to discover such representations as it sees fit. Every piece of design which goes into a module of the system or the ways in which modules are connected reduces that freedom. However, modular design can make the learning process tractable and flexible, and dramatically reduce the need for training data.
Building in the right assumptions
There are certain characteristics of the real world that Spatial AI systems must work in that seem so elementary that it is unnecessary to spend training capacity on learning them. These could include:
- Basic geometry of 3D transformation as a camera sees the world from different views
- Physics of how objects fall and interact
- The simple fact that the natural world is made up of separable objects at all
- Environments are made up of many objects in configurations with a typical range of variability over time which can be estimated and mapped.
By building these and other assumptions into modular estimation frameworks that still have significant deep learning capacity in the areas of both semantics and geometrical estimation, we believe that we can make rapid progress towards highly capable and adaptable Spatial AI systems. Modular systems have the further key advantage over purely learned methods that they can be inspected, debugged and controlled by their human users, which is key to the reliability and safety of products.
We still believe fundamentally in Spatial AI as a SLAM problem, and that a recognizable mapping capability will be the key to enabling robots and other intelligent devices to perform complicated, multi-stage tasks in their environments.
For those who want to read more about this area, please see my paper “FutureMapping: The Computational Structure of Spatial AI Systems.”
About the Author
Professor Andrew Davison is a co-founder of SLAMcore, a London-based company that is on a mission to make spatial AI accessible to all. SLAMcore develops algorithms that help robots and drones understand where they are and what’s around them – in an affordable way.
Davison is Professor of Robot Vision at the Department of Computing, Imperial College London and leads Imperial’s Robot Vision Research Group has spent 20 years conducting pioneering research in visual SLAM, with a particular emphasis on methods that work in real-time with commodity cameras.
He has developed and collaborated on breakthrough SLAM systems including MonoSLAM and KinectFusion, and his research contributions have over 15,000 academic citations. He also has extensive experience of collaborating with industry on the application of SLAM methods to real products.