随着人工智能技术向军事领域的广泛渗透,并逐步物化或赋能武器装备,部队“机器人换人”加速,人机比例持续下降,编成模式向模块化编组、积木式组合和任务式联合方向...
哈佛大学升级Kirigani蛇形机器人 特殊外形纹理改善速度和准确度
机器人粒子模拟系统脱离概念 它可能会为你捏寿司哦!
Drone delivery taking off from Alphabet’s Wing Aviation
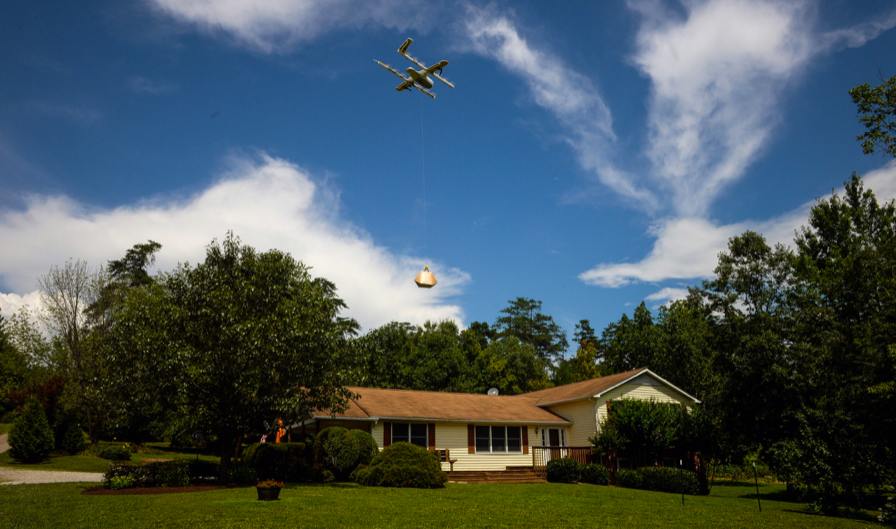
A Wing Aviation drone delivers a package to a home during a demo in Blacksburg, Virginia. | Credit: Bloomberg
Alphabet Inc. subsidiary Wing Aviation on Tuesday became the first drone delivery company to be awarded air carrier certification from the Federal Aviation Administration (FAA). With the certification, Wing Aviation now has the same certifications as smaller airlines and can turn its tests into a commercial service that delivers goods from local businesses to homes.
The approval grants Wing permission to conduct flights beyond visual line of sight and over people, Wing says. The company will start commercial deliveries in Blacksburg, Virginia later in 2019. Wing spun out of Alphabet’s X research division in July 2018.
“This is an important step forward for the safe testing and integration of drones into our economy,” said U.S. Secretary of Transportation, Elaine L. Chao, who made the announcement. “Safety continues to be our Number One priority as this technology continues to develop and realize its full potential.”
Part of the approval process required Wing Aviation to submit evidence that its operations are safe. Wing’s drones have flown more than 70,000 test flights and made more than 3,000 deliveries. Wing says it submitted data that shows “delivery by Wing carries a lower risk to pedestrians than the same trip made by car.”
PwC estimates the total addressable market for commercial drones is $127.3 billion. That includes $45.2 billion in infrastructure, $32.4 billion in agriculture, $13 billion in transport and $10.5 billion in security.
Wing’s electric drones are powered by 14 propellers and can carry loads of up to 1.5 kilograms (3.3 pounds). Wing’s drones can fly up to 120 kilometers (about 74.5 miles) per hour and can fly up to 400 feet above the ground. Wing’s drones convert GPS signals into latitude and longitude to determine location and speed.
The drones also have a number of redundant systems on board for operation and navigation, among them a downward-facing camera used as a backup to GPS navigation. If the GPS is unavailable for any reason, the drone uses data from the camera to measure speed, latitude and longitude in its place. The camera is used exclusively for navigation, it doesn’t capture video and is not available in real time.
Drone regulations still don’t permit most flights over crowds and urban areas. This will, of course, limit where Wing can operate. But the company said it plans to start charging soon for deliveries in Blacksburg and eventually apply for permission to expand to other regions.
The first of Wing’s drone deliveries were completed in 2014 in Queensland, Australia, where everything from dog treats to a first-aid kit were delivered to farmers. Two years later, Wing’s drones delivered burritos to Virginia Tech students. “Goods like medicine or food can now be delivered faster by drone, giving families, shift workers, and other busy consumers more time to do the things that matter,” Wing Aviation writes in a blog. “Air delivery also provides greater autonomy to those who need assistance with mobility.”
Just a couple weeks prior to the FAA certification, Wing made its first drone delivery in Cranberry, Australia after recently receiving approval from the country’s Civil Aviation Authority. To start, Wing service will be available to 100 homes and will be slowly expanded to other customers.
Wing was first known as “Project Wing” when it was introduced in 2014. Google X made the announcement via the video below, which shows early test flights in Queensland.
Wing is also launching its first European drone delivery service in Finland this spring. In its tests in Australia, the average Wing delivery was completed in 7 minutes 36 seconds, according to a spokeswoman.
In June 2016, Wing worked with NASA and the FAA to explore how to manage drones. Wing demonstrated its Unmanned Traffic Management (UTM), real-time route planning, and airspace notifications. Wing’s UTM platform is designed to support the growing drone industry by enabling a high volume of drones to share the skies and fly safely over people, around terrain and buildings, and near airports, which remain hurdles before drone deliveries become commonplace in the US. A DJI drone was recently spotted illegally flying over Fenway Park where a ban should have been in place.
Wing says on its website, “we’re working with the FAA on the Low Altitude Authorization and Notification Capability (LAANC) system in the United States and with the Civil Aviation Safety Authority (CASA) in Australia to develop federated, industry-led solutions to safely integrate and manage drones in low-altitude airspace.”
波士顿动力计划今年生产约100台SpotMini
特斯拉将推自动驾驶出租车 未来将去掉方向盘和脚踏板
Robotic catheter brings autonomous navigation into human body
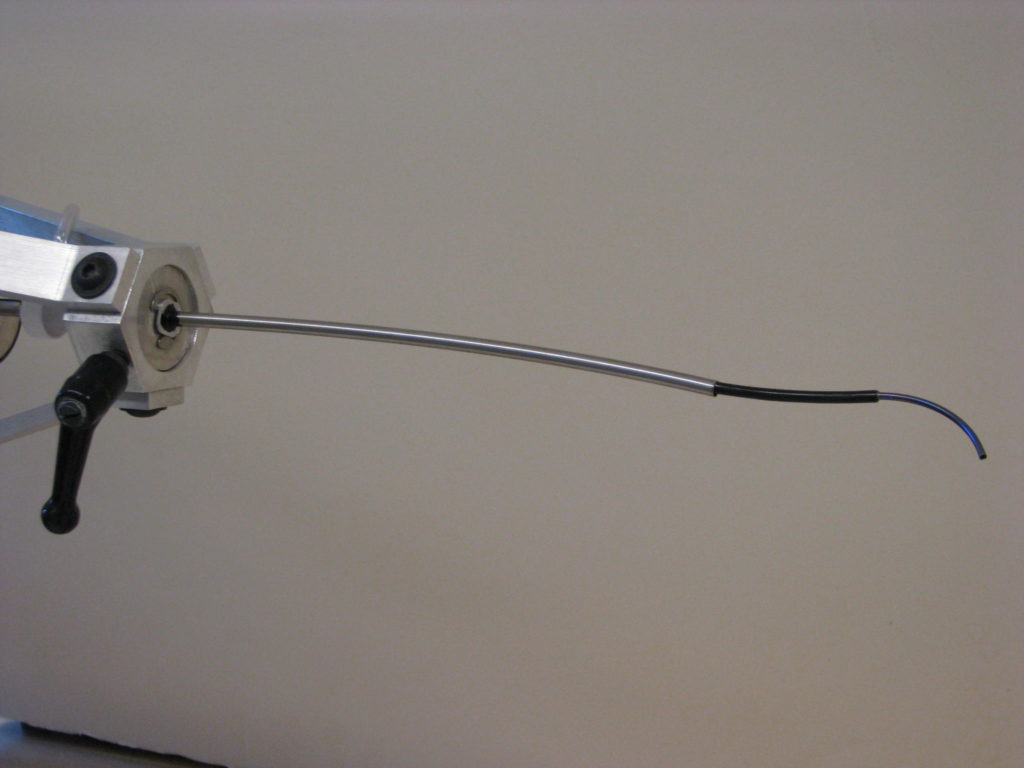
Concentric tube robot. In a recent demo, robotic catheter autonomously found its way to a leaky heart valve. Source: Pediatric Cardiac Bioengineering Lab, Department of Cardiovascular Surgery, Boston Children’s Hospital, Harvard Medical School
BOSTON — Bioengineers at Boston Children’s Hospital said they successfully demonstrated for the first time a robot able to navigate autonomously inside the body. In a live pig, the team programmed a robotic catheter to find its way along the walls of a beating, blood-filled heart to a leaky valve — without a surgeon’s guidance. They reported their work today in Science Robotics.
Surgeons have used robots operated by joysticks for more than a decade, and teams have shown that tiny robots can be steered through the body by external forces such as magnetism. However, senior investigator Pierre Dupont, Ph.D., chief of Pediatric Cardiac Bioengineering at Boston Children’s, said that to his knowledge, this is the first report of the equivalent of a self-driving car navigating to a desired destination inside the body.
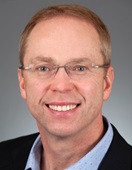
Pierre Dupont, chief of Pediatric Cardiac Bioengieering at Boston Children’s Hospital
Dupont said he envisions autonomous robots assisting surgeons in complex operations, reducing fatigue and freeing surgeons to focus on the most difficult maneuvers, improving outcomes.
“The right way to think about this is through the analogy of a fighter pilot and a fighter plane,” he said. “The fighter plane takes on the routine tasks like flying the plane, so the pilot can focus on the higher-level tasks of the mission.”
Touch-guided vision, informed by AI
The team’s robotic catheter navigated using an optical touch sensor developed in Dupont’s lab, informed by a map of the cardiac anatomy and preoperative scans. The touch sensor uses artificial intelligence and image processing algorithms to enable the catheter to figure out where it is in the heart and where it needs to go.
For the demo, the team performed a highly technically demanding procedure known as paravalvular aortic leak closure, which repairs replacement heart valves that have begun leaking around the edges. (The team constructed its own valves for the experiments.) Once the robotic catheter reached the leak location, an experienced cardiac surgeon took control and inserted a plug to close the leak.
In repeated trials, the robotic catheter successfully navigated to heart valve leaks in roughly the same amount of time as the surgeon (using either a hand tool or a joystick-controlled robot).
Biologically inspired navigation
Through a navigational technique called “wall following,” the robotic catheter’s optical touch sensor sampled its environment at regular intervals, in much the way insects’ antennae or the whiskers of rodents sample their surroundings to build mental maps of unfamiliar, dark environments. The sensor told the catheter whether it was touching blood, the heart wall or a valve (through images from a tip-mounted camera) and how hard it was pressing (to keep it from damaging the beating heart).
Data from preoperative imaging and machine learning algorithms helped the catheter interpret visual features. In this way, the robotic catheter advanced by itself from the base of the heart, along the wall of the left ventricle and around the leaky valve until it reached the location of the leak.
“The algorithms help the catheter figure out what type of tissue it’s touching, where it is in the heart, and how it should choose its next motion to get where we want it to go,” Dupont explained.
Though the autonomous robot took a bit longer than the surgeon to reach the leaky valve, its wall-following technique meant that it took the longest path.
“The navigation time was statistically equivalent for all, which we think is pretty impressive given that you’re inside the blood-filled beating heart and trying to reach a millimeter-scale target on a specific valve,” said Dupont.
He added that the robot’s ability to visualize and sense its environment could eliminate the need for fluoroscopic imaging, which is typically used in this operation and exposes patients to ionizing radiation.
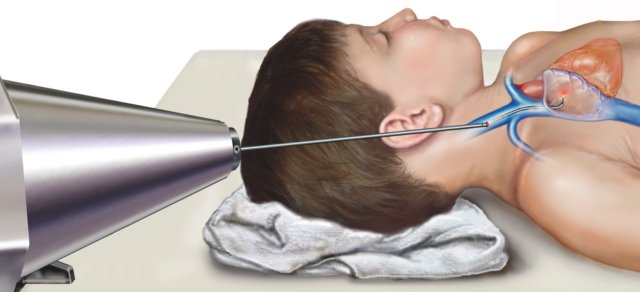
Robotic catheter enters internal jugular vein and navigates through the vasculature into the right atrium. Source: Pediatric Cardiac Bioengineering Lab
A vision of the future?
Dupont said the project was the most challenging of his career. While the cardiac surgical fellow, who performed the operations on swine, was able to relax while the robot found the valve leaks, the project was taxing for Dupont’s engineering fellows, who sometimes had to reprogram the robot mid-operation as they perfected the technology.
“I remember times when the engineers on our team walked out of the OR completely exhausted, but we managed to pull it off,” said Dupont. “Now that we’ve demonstrated autonomous navigation, much more is possible.”
Some cardiac interventionalists who are aware of Dupont’s work envision using robots for more than navigation, performing routine heart-mapping tasks, for example. Some envision this technology providing guidance during particularly difficult or unusual cases or assisting in operations in parts of the world that lack highly experienced surgeons.
As the U.S. Food and Drug Administration begins to develop a regulatory framework for AI-enabled devices, Dupont said that autonomous surgical robots all over the world could pool their data to continuously improve performance over time — much like self-driving vehicles in the field send their data back to Tesla to refine its algorithms.
“This would not only level the playing field, it would raise it,” said Dupont. “Every clinician in the world would be operating at a level of skill and experience equivalent to the best in their field. This has always been the promise of medical robots. Autonomy may be what gets us there.”
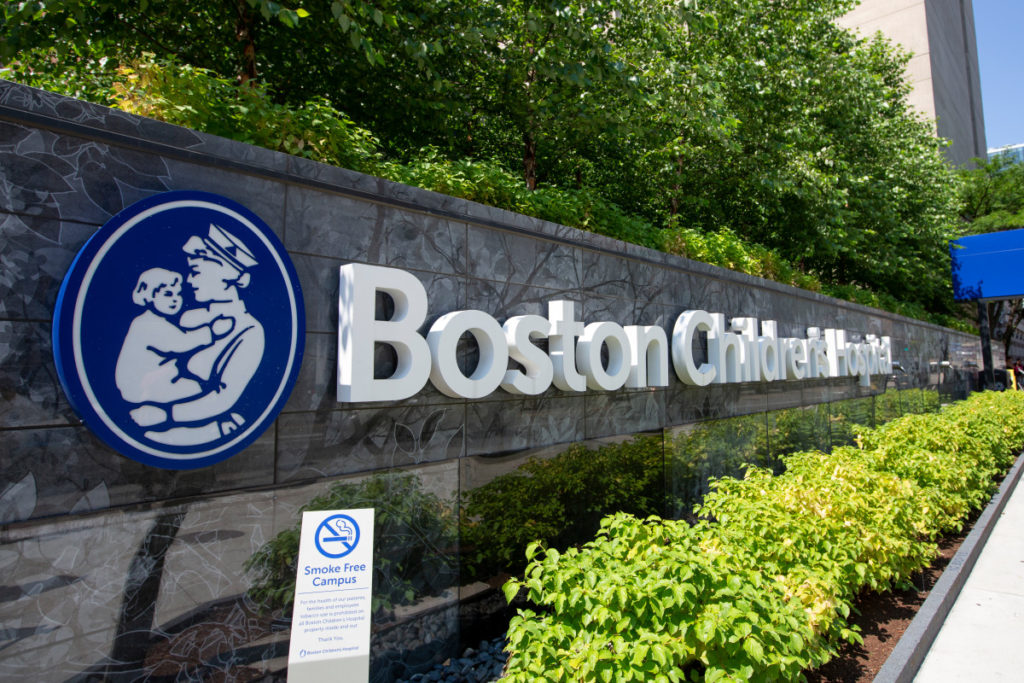
Boston Children’s Hospital in the Longwood Medical Area. Photo by Jenna Lang.
About the paper
Georgios Fagogenis, PhD, of Boston Children’s Hospital was first author on the paper. Coauthors were Margherita Mencattelli, PhD, Zurab Machaidze, MD, Karl Price, MaSC, Viktoria Weixler, MD, Mossab Saeed, MB, BS, and John Mayer, MD of Boston Children’s Hospital; Benoit Rosa, PhD, of ICube, Universite? de Strasbourg (Strasbourg, France); and Fei-Yi Wu, MD, of Taipei Veterans General Hospital, Taipei, Taiwan. For more on the technology, contact TIDO@childrenshospital.org.
The study was funded by the National Institutes of Health (R01HL124020), with partial support from the ANR/Investissement d’avenir program. Dupont and several of his coauthors are inventors on U.S. patent application held by Boston Children’s Hospital that covers the optical imaging technique.
About Boston Children’s Hospital
Boston Children’s Hospital, the primary pediatric teaching affiliate of Harvard Medical School, said it is home to the world’s largest research enterprise based at a pediatric medical center. Its discoveries have benefited both children and adults since 1869. Today, more than 3,000 scientists, including 8 members of the National Academy of Sciences, 18 members of the National Academy of Medicine and 12 Howard Hughes Medical Investigators comprise Boston Children’s research community.
Founded as a 20-bed hospital for children, Boston Children’s is now a 415-bed comprehensive center for pediatric and adolescent health care. For more, visit the Vector and Thriving blogs and follow it on social media @BostonChildrens, @BCH_Innovation, Facebook and YouTube.
Neural network helps autonomous car learn to handle the unknown
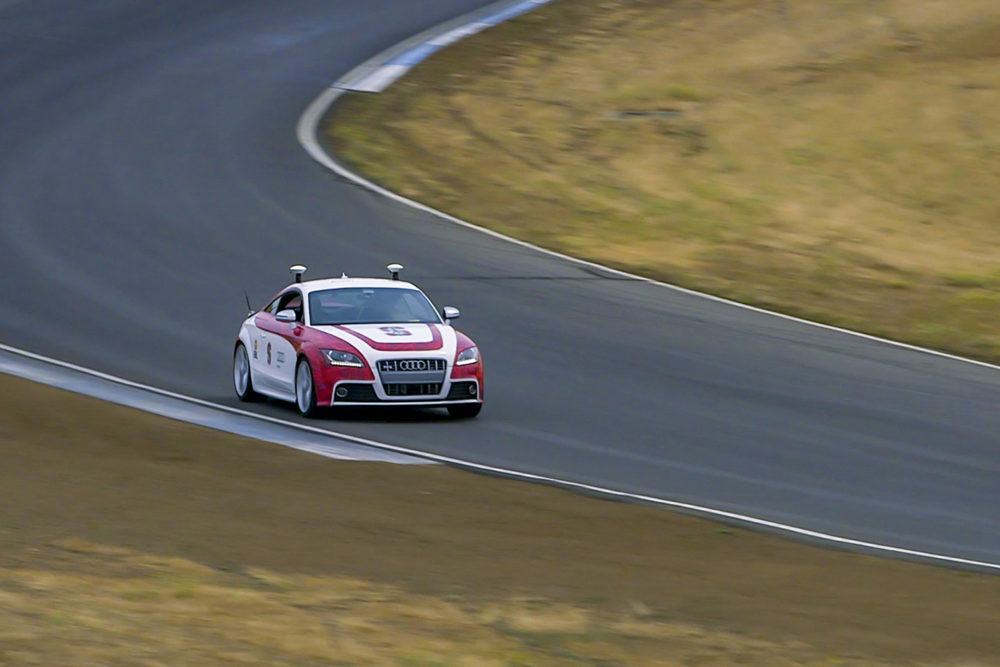
Shelley, Stanford’s autonomous Audi TTS, performs at Thunderhill Raceway Park. (Credit: Kurt Hickman)
Researchers at Stanford University have developed a new way of controlling autonomous cars that integrates prior driving experiences – a system that will help the cars perform more safely in extreme and unknown circumstances. Tested at the limits of friction on a racetrack using Niki, Stanford’s autonomous Volkswagen GTI, and Shelley, Stanford’s autonomous Audi TTS, the system performed about as well as an existing autonomous control system and an experienced racecar driver.
“Our work is motivated by safety, and we want autonomous vehicles to work in many scenarios, from normal driving on high-friction asphalt to fast, low-friction driving in ice and snow,” said Nathan Spielberg, a graduate student in mechanical engineering at Stanford and lead author of the paper about this research, published March 27 in Science Robotics. “We want our algorithms to be as good as the best skilled drivers—and, hopefully, better.”
While current autonomous cars might rely on in-the-moment evaluations of their environment, the control system these researchers designed incorporates data from recent maneuvers and past driving experiences – including trips Niki took around an icy test track near the Arctic Circle. Its ability to learn from the past could prove particularly powerful, given the abundance of autonomous car data researchers are producing in the process of developing these vehicles.
Physics and learning with a neural network
Control systems for autonomous cars need access to information about the available road-tire friction. This information dictates the limits of how hard the car can brake, accelerate and steer in order to stay on the road in critical emergency scenarios. If engineers want to safely push an autonomous car to its limits, such as having it plan an emergency maneuver on ice, they have to provide it with details, like the road-tire friction, in advance. This is difficult in the real world where friction is variable and often is difficult to predict.
To develop a more flexible, responsive control system, the researchers built a neural network that integrates data from past driving experiences at Thunderhill Raceway in Willows, California, and a winter test facility with foundational knowledge provided by 200,000 physics-based trajectories.
This video above shows the neural network controller implemented on an automated autonomous Volkswagen GTI tested at the limits of handling (the ability of a vehicle to maneuver a track or road without skidding out of control) at Thunderhill Raceway.
“With the techniques available today, you often have to choose between data-driven methods and approaches grounded in fundamental physics,” said J. Christian Gerdes, professor of mechanical engineering and senior author of the paper. “We think the path forward is to blend these approaches in order to harness their individual strengths. Physics can provide insight into structuring and validating neural network models that, in turn, can leverage massive amounts of data.”
The group ran comparison tests for their new system at Thunderhill Raceway. First, Shelley sped around controlled by the physics-based autonomous system, pre-loaded with set information about the course and conditions. When compared on the same course during 10 consecutive trials, Shelley and a skilled amateur driver generated comparable lap times. Then, the researchers loaded Niki with their new neural network system. The car performed similarly running both the learned and physics-based systems, even though the neural network lacked explicit information about road friction.
In simulated tests, the neural network system outperformed the physics-based system in both high-friction and low-friction scenarios. It did particularly well in scenarios that mixed those two conditions.
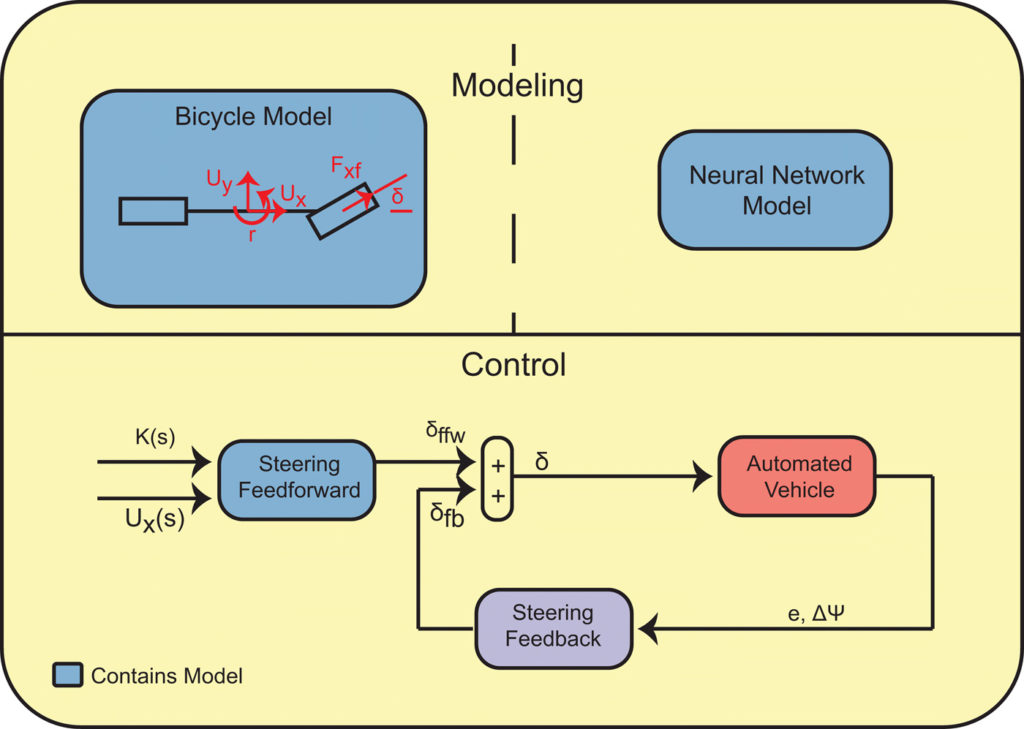
Simple feedforward-feedback control structure used for path tracking on an automated vehicle. (Credit: Stanford University)
An abundance of data
The results were encouraging, but the researchers stress that their neural network system does not perform well in conditions outside the ones it has experienced. They say as autonomous cars generate additional data to train their network, the cars should be able to handle a wider range of conditions.
“With so many self-driving cars on the roads and in development, there is an abundance of data being generated from all kinds of driving scenarios,” Spielberg said. “We wanted to build a neural network because there should be some way to make use of that data. If we can develop vehicles that have seen thousands of times more interactions than we have, we can hopefully make them safer.”
Editor’s Note: This article was republished from Stanford University.
The post Neural network helps autonomous car learn to handle the unknown appeared first on The Robot Report.